After Sora’s official launch, another hot technology track, embodied intelligence, has recently ushered in the commercialization “eve”.
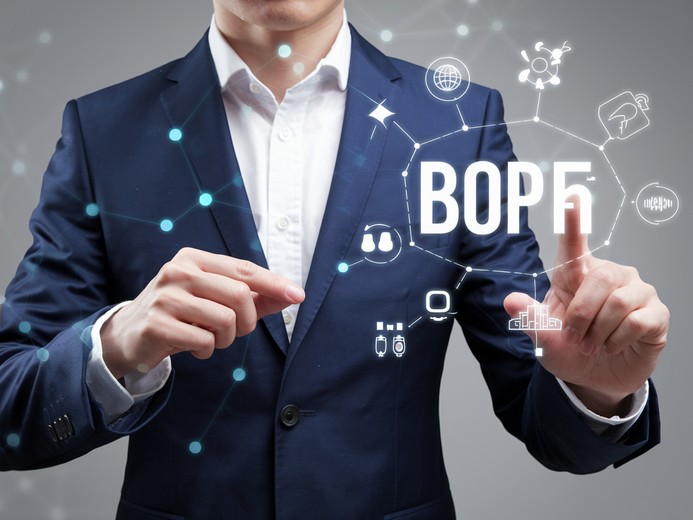
On December 16, 2024, Zhiyuan Robotics, founded by “Huawei Boy Genius” and “Zhihuijun” (Peng Zhihui), said to China tenology headhunter SunTzu Recruit that it has commenced the mass production of general-purpose robots. This comes just four months after Zhiyuan Robotics released five new commercial humanoid robots from its “Yuanzheng” and “Lingxi” families.
At nearly the same time, the China Embodied Intelligence Headhunter noted that BYD official Weibo released a recruitment post for the personified intelligent direction, facing the 2025 class of global universities, recruiting masters and Ph.D. graduates to the personified intelligent research team to promote the industrial application of personified intelligence. Xiaomi Group’s core founding team founded the large model robot company – Xiaoyu Zhizao, which recently completed a new round of financing to increase R&D efforts and promote the innovation of personified intelligent products.
Looking back, this year’s various exhibitions have become a stage for embodied intelligence to “show its muscles”, and people are imagining the future in scenes of human-machine coexistence. However, back to reality, with the advent of commercialization, embodied intelligence faces more challenges from the three-dimensional world compared to the “large model” of human-computer interaction.
From “good-looking skin” to “useful carrier”
At the exhibition, China humanoid headhunter saw humanoid robots danced, interacted, sorted items, and wiped tables on the spot, becoming the “secret weapon” to attract the audience. If the large model is likened to an “interesting soul”, embodied intelligence is a “beautiful skin”. However, apart from exhibitions, there are not many scenarios where embodied intelligence is actually implemented. Data is the core of how to make embodied intelligence transition from a “beautiful skin” to a “useful carrier”.
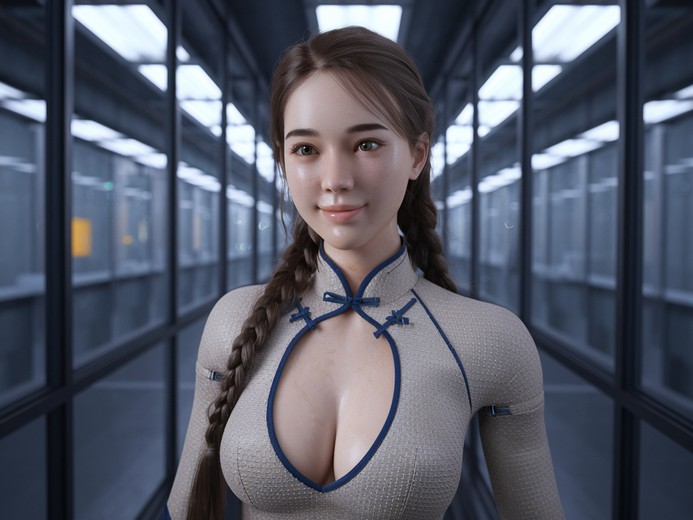
“The biggest difference we’ve found in the development of embodied intelligence and multi-modal large models is the scarcity of robot data,” said Yao Maoqing, President of Embodied Business Unit at Yuan Zhixin, at the Embodied Intelligence Special Forum of the Pujiang AI Academic Conference. Compared with large models that can obtain free Internet data, the highest quality, labeled data that robots can use is only a few million entries in a data set, and these data sets are a mixture of multiple formats with uneven quality. “Therefore, the most common demos we see are only desktop operations of humanoid robots, such as moving fruits and blocks around.”
Smart Yuan Robots
“The datasets of physical world available are far from enough, and embodied intelligence is still in its early stage.” Zhou Bin, vice president of Shanghai Fourier, added that manual remote operation is the current mainstream method of robot data collection, and its core goal is to make the operation of robot body as close to human behavior patterns as possible. However, this method requires a lot of manpower and time.
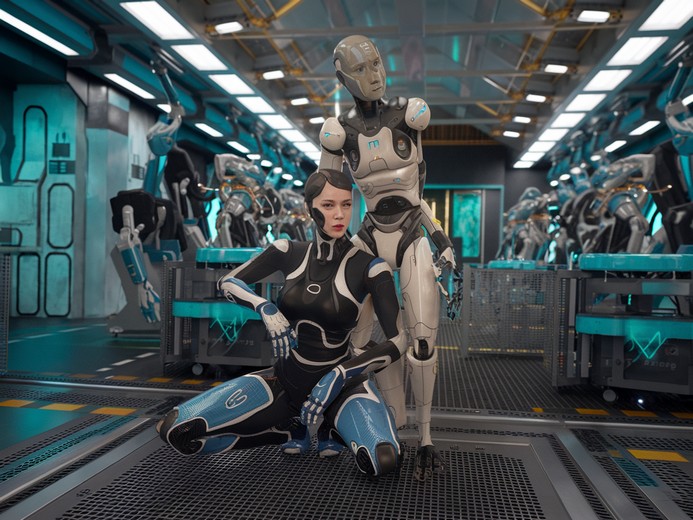
As an example, Tesla’s recruitment website shows that “data collection operator” has the highest hourly wage of 48 US dollars. In addition to wearing motion capture suit and VR headgear for a long time, they also need to walk for more than 7 hours a day, and work in three shifts, so that robots can continuously absorb data for 24 hours.
Another way of data collection is by combining the virtual with the real. This method requires the collection of data from the physical world first, and then the generation of synthetic data. The advantage is that it is fast to obtain and the cost is low, but the disadvantage is that it can only support embodied intelligence to perform simple movements such as walking and jumping. When facing more realistic and complex environments, the demand for computing resources and data volume increases exponentially.
Because the application scenarios of embodied intelligence are extremely extensive, involving multiple modalities, different formats, and different scales of data, the data ecosystem of embodied intelligence is very important. Zhang Zhaoxiang, a researcher at the Institute of Automation, Chinese Academy of Sciences, believes that it is necessary to establish a unified data framework at the ecological level. Fang Bin, a professor at Beijing University of Posts and Telecommunications, also said that the industry, academia, and enterprises need to form a joint force on data.
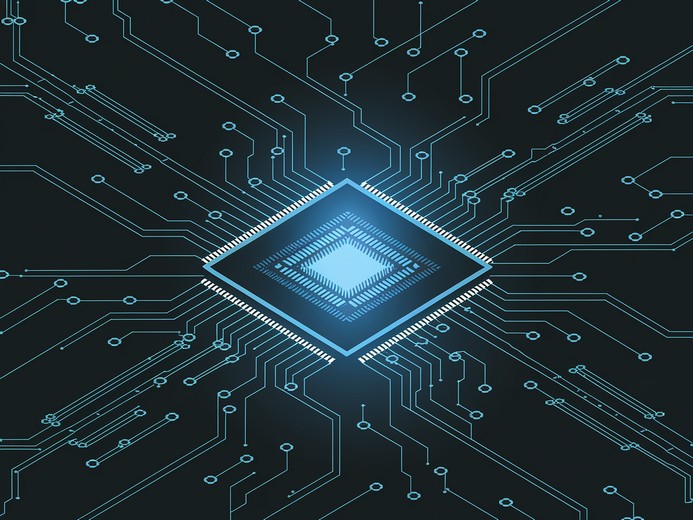
Fortunately, this year, many companies have open-sourced and begun to build high-quality embodied intelligence datasets. For example, the National and Local Co-built Humanoid Robot Innovation Center has created the Openloong open-source community, which uses the community and training ground innovation mechanisms to accelerate the work of humanoid robots, embodied intelligence training, and dataset construction; the Beijing Institute of Embodied Intelligent Robots has also started the construction of embodied intelligent datasets and data application platforms; the Pengcheng Laboratory Multi-intelligence and Embodied Intelligence Institute has jointly released and open-source the embodied large-scale dataset ARIO with many universities.
Sora can be used as a reference object
After Sora’s official launch, most users were amazed by its powerful consistency control capabilities. However, this does not mean that Sora is perfect. After a week of evaluation, foreign technology blogger Marques Brownlee stated that Sora’s understanding of physical laws is not good enough, and there are still situations where people’s hands are unnatural, text is garbled, and animals fly while running.
China humanoid headhunter believes whether it is a generative large model or embodied intelligence, robots can only make decisions and execute complex tasks by accurately perceiving and understanding the 3D physical world.
Humanoid robot Atlas
A domestic text-to-video generation company said in an interview with the media that videos are composed of frame-by-frame images arranged in order, and this principle is an important path for embodied intelligence to understand the world. The principle is to allow robots to learn continuous images and obtain rich information about objects and environments from them, so as to deepen the robots’ understanding.
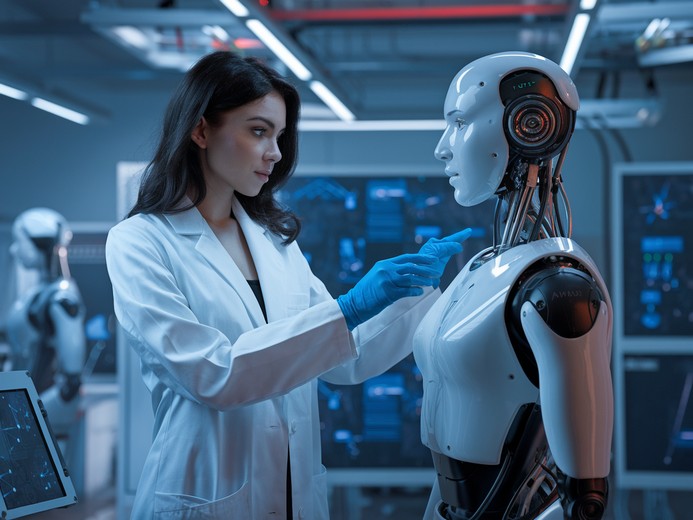
The specific solution is to first collect data through video websites, then feed the video generation results back into the embodied intelligence, while mechanical and other physical world data collection serves as a supplementary method.
Another approach proposed by Huang Siyuan, a scientist at the Beijing Academy of General Artificial Intelligence, is the “brain+cerebellum”, in which a large model serves as the main control, with multiple small models connected to it to decompose the entire task into specific steps. Each step is associated with scene objects, ensuring that the model’s output is based on specific information from the real world.
In terms of training, Huang Siyuan said that it can be divided into two parts: the first step is to align enough 3D data with descriptions; the second step is to use higher-level data pre-training to train higher-level tasks. “Higher-level data” typically refers to data that requires higher-level understanding and processing, such as complex scene understanding and behavior prediction, which are often more abstract and require stronger reasoning and comprehension abilities.
Visuotactile: Making Embodied Intelligence “Full of Blood and Flesh”
For embodied intelligence, data grants a “soul”, a large model grants “wisdom”, and actuators enable the capability of action. Moreover, actuators offer the most intuitive expression of embodied intelligence’s interactive abilities.
However, as of now, there is still a gap between “doing the will” of embodied intelligence.
Position control and force control are the two main ways for embodied intelligence to grasp objects.
Yang Zhengye, director of market system of National Center for Geographic Information, once told the reporter of IT Times that position control is that the robot calculates the volume or size of the object first, and then moves the fingers to the spatial position when grasping. Once the calculation deviates, it will lead to two consequences: one is that the fingers will be broken to reach the position, and the other is that the object will be directly damaged.
Power control is the analysis of how much force is needed to grasp an object. Even if there is a deviation, it can reduce or even prevent the occurrence of the above two situations. This requires the embodiment of intelligence with visual tactile perception capabilities.
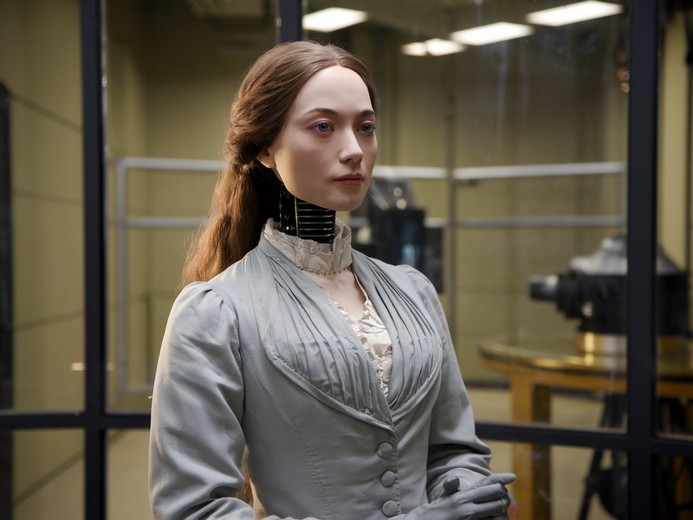
Professor Fang Bin of Beijing University of Posts and Telecommunications introduced the principle of tactile vision, which can be understood as expressing tactile sensation based on images. That is, after tactile sensor obtains tactile data, it is converted into image form, and the format is consistent with the image information captured by visual camera, which makes data processing and analysis more efficient.
However, Fang Bin believes that tactile perception is more personalized than vision. “People’s visual perception is similar, but their tactile perception can vary.” Therefore, the key to improving tactile ability lies in contact-based operation. However, traditional tactile sensors provide contact data under the combined force state. When facing complex operations, a single combination force is difficult to complete the task, especially when facing some flexible operations.
Therefore, Fang Bin’s team created the Tacchi visual-tactile simulator, which not only provides single-press responses but also simulates various motion patterns such as slight sliding and rotation when touching an object, making the tactile information of the tactile sensor more accurate. “In the future, we hope to apply the simulator to visual-tactile sensors of various shapes and break the status quo of only visual modalities.”
Comments are closed